Abstract
In Indian Navy, multiple organisations interact with each other for a common goal: combat ready ships. Each organisation works independently for its defined charter of duties and interacts administratively with each other. It is pertinent to observe that although each organisation works independently, their mutual interaction with each other is a highly complex phenomenon akin to multiple heavy machinery interacting with each other in a complex engineering system. In some of the organisations like naval dockyards and shipbuilding yards, where ships are refitted/ built, Indian Navy has migrated to Enterprise Resource Planning (ERP) where the interaction of individual departments of dockyard is co-ordinated using software-based tools to optimise efficiency. However, most of these ERP systems used in Navy are intra-organisational. Sub departments within the organisation interact through these ERP systems simplifying the complexity and interdependency of the multi-faceted nature of the organisation. A similar characteristic is relevant for the jointmanship of the three services. This paper goes a step beyond and proposes an inter organisational ERP based model. This proposed IT tool would serve to achieve administrative co-ordination between multiple organisations of Indian Navy and other sister services, as well as, will aid in decision making of each organisation based on various kinds of maintenance data of their assets that would be analysed in a Reliability Centric Maintenance generated ecosystem. Therefore, a three pronged, comprehensive RCMbased inter-organisational ERP model for optimised efficiency and performance of tri-services is proposed herewith. The first step is a digital data and machine learning-based reliability monitoring system onboard naval platforms, as well as for similar assets of the army and airforce. The second step is transferring all the digital data to a central data processing centre ashore. The third step is integrating data from repair yards and logistic services to deliver a comprehensive process optimisation solution. This data-based ERP model would work independently at the lowest component level through equipment level, system level, ship/unit level, fleet/ formation level, command/ brigade level to the highest level of command and control.1
Introduction
In the modern era, the performance of war machinery plays a bigger role than leadership and strategy, towards the success of any conflict. Currently, the Indian Navy relies on condition monitoring tools for Condition Based Predictive Maintenance, as the maintenance philosophy which drives the operational cycle of the ships. This operational cycle defines the work for repair yards and logisticians to plan their charter of duties and inventory schedule respectively. There are two basic challenges in this workflow. The first is in the CBPM based maintenance philosophy and the second challenge is lack of databased decision support system for mutual interaction amongst these organisations towards optimal availability of assets, which in turn determine the combat capability of the naval force. Similar, situation is perhaps relevant for assets of other sister services.
Bottlenecks in CBPM and ERP
There are many state-of-the-art CBPM tools presently in use in Indian Navy. These CBPM tools {like Narrowband analysis, trend analysis, lub oil particle analysis or Unified Maintenance Management System based reliability monitoring tool, like NETRA} depend on the manually logged data. Manual logging has inherent problems like erroneous entry, undefined sampling rate, loss of data etc. The manual data is also not situation-aware. Before dwelling any further to discuss the merits and demerits of manually logged data, it is important to define the term “Situation Awareness”. The concept of ‘Situation-Aware’ is being increasingly used in data analytics and human factor circles. The concept of situation awareness is explained further.2
Situation Awareness
A Challenger II tank, engaged in defending a bridge over the Shatt al Basra canal on the western outskirts of Basra, fired upon what its Commander believed were enemy personnel moving in and out of an ammunition bunker. Unknown to the Commander, the engaged target was actually two friendly Challenger II tanks from another squadron, sited in an overwatch position adjacent to a dam only 1,500 metres to the southeast of his own position. The first High Explosive Squash Head (HESH) round fired landed short but the effects of the blast were sufficient to throw the crew members from the tanks’ turrets. The second round was a direct hit, detonating in the Commander’s hatch of one of the Challenger tanks, killing its two occupants instantly. The incident described is an extreme example of what can happen in a complex sociotechnical system when the operators working within the system are not fully cognisant of everything that they need to know. In the aftermath, the official government inquiry (Ministry of Defence, 2004) identified various causal factors, including a lack of what is called Situation Awareness (SA) on behalf of those involved. SA is the term that is used within Human Factors (HF) and ergonomics circles to describe the level of awareness that people have of the situation that they are engaged in. It focuses on how people develop and maintain a sufficient understanding of ‘what is going on’ and what is likely to go on in order to achieve success in task performance.
Safe and efficient task performance within complex sociotechnical systems depends on organisations acquiring and maintaining appropriate levels of SA. Systems, devices and procedures therefore need to be designed so that they facilitate, rather than inhibit, SA acquisition and maintenance. Designing systems in this manner depends on the accurate description of how SA operates in the system in question, on exactly what information SA comprises during task performance and on how this information is integrated and used by different enterprises working within the system. Further, reliable and valid approaches for modelling SA are required in order to determine how a new system, device, training programme and procedural design affect SA during operations.
Having understood what is SA in our context of enterprise resource planning, let us return to the original discussion about the data that we use for monitoring our equipment and systems being not situation-aware and how this affects our decision making and efficiency. The inescapable conclusions from this understanding of SA are that currently there is a lack of an appropriate model of ‘Situational Awareness’ (SA) for complex collaborative environments and also that existing SA assessment methods are inadequate when considering the measurement of SA during real-world collaborative activities.
These observations of monitoring or being situation-aware is not at an individual organisational level but at a complex system of systems level where each individual organisation is interacting with each other and therefore giving us an emergent behaviour which is an output of complex interactions. This complex interaction of individual enterprises confirm the assumption that our understanding of SA in such environments remain limited and subsequently serve to set the agenda for the rest of the paper; that is, it is our aim to further investigate the description and measurement of SA in collaborative environments. Our lack of knowledge regarding enterprise-level SA acquisition and maintenance and the accompanying lack of approaches for measuring team SA is the issue that is being addressed by this paper.
Considering the bottlenecks in our present processes and systems, the current proposal is a solution aided by the digital data-based RCM philosophy and how this digital RCM will aid in enterprise-level decision making.
Proposed Solution.
A Distributed Situational Awareness (DSA) model, accounts for SA in collaborative environments. Following this, the propositional networks methodology – an RCM based modelling approach that can be used to describe and assess DSA during real world collaborative tasks – is described. A simple command and control paradigm example is then used to demonstrate both approaches.
Distributed Situational Awareness
- Data on the Network. The present-day Indian Naval Ships are being commissioned with Integrated Platform Management Systems (IPMS). All the equipment, machinery, systems, system of systems are connected to a common network called IPMS. All the assets of a ship are sharing their running parameters on this IPMS network. The running parameters of each equipment are being logged in both digital (Unlabelled data) and CSV format . This data can profusely aid in developing fleet level, system level and equipment level situational awareness. This data in the IPMS can be collected in three ways.
- E- Logging. On those ships where the data is being logged in CSV format, the data can be directly taken through any suitable hard disk format or through NUD. The challenge with this kind of data extraction is that the data cannot be taken when the ship is sailing. The intermittent logging of data will not support the machine learning-based condition monitoring models or the RCM models.
- Digital Data. The second option is to directly transfer the sensor-based digital data through the Navy’s own satellite bandwidth. The digital data from the IPMS would be encrypted and compressed and then transferred to a shore-based data processing centre. At the data processing centre, this data will be decrypted and decompressed for further processing and labelling of data. The labelled data can be further fed into the machine learning-based reliability assessment models.
- Digital Data without Access to Network Protocol. A major bottleneck in the data extraction would emerge if the OEMs of the IPMS do not share the network protocol. If the network protocol is not provided to the end user under proprietary laws, then we can harness the data by developing a software patch to extract the data. Using this patch, we can break into the network protocol of any IPMS-based platform and utilise the data.
- Data Transfer to a Reliability Assessment Centre. The data that is collected from the IPMS platforms would have to be compressed and encrypted before it could be transferred to a data processing centre or reliability assessment centre. For compression of the data, a collaborative effort is underway with M/s CDAC which holds a patent for a supercomputer that can compress the data by upto 10X. The compression of data by 10X will have two benefits. The first benefit would be in utilising only a limited bandwidth of the satellite communication system for transferring the data. The second benefit would be improved sampling frequency. Improved sampling frequency would give a better result out of the reliability assessment models. After the data is transferred to a reliability assessment centre, the data would be decompressed and decrypted. The data labelling and data processing would be done on the original data after decompression and decryption. The processed data would now be integrated with data from Material Organisation, which provides information on inventory consumption and from dockyard/ repair yard which provides maintenance data, for feeding into the machine learning-based reliability assessment model.
- Data Integration. Data integration is a crucial component for enhancing material and inventory management through situational awareness and reliability-centred maintenance. It involves the aggregation and harmonization of data from various sources to provide a unified, comprehensive view of inventory status, maintenance requirements, and operational conditions. Effective data integration supports informed decision-making, optimizes resource allocation, and enhances overall operational efficiency. The data taken from IPMS-based ships will generate only ship-level situation awareness. To derive any meaningful value addition in the enterprise-level situation awareness, data from the individual organisations like Material Organisation, Naval Dockyard, MTU, INSMA etc has to be integrated with the IPMS-based data from ships.3
- Key Elements of Data from Dockyard and Material Organisation4
- Supply Chain Databases. Capturing data on procurement of equipment parts, time taken in effective shipment from the vendor and bureaucratic delays in receiving the item from the time demand is raised and inventory levels from material organisation etc. This data can be captured from ERP software tools like ILMS and transferred to the data processing centre.
- Maintenance Logs. Gathering historical and real-time data from maintenance records, including details on repairs, part replacements, and equipment performance has to be extracted from the Naval Dockyards for integration with MO and Ship data.
- Operational Reports. Data from daily maintenance operations like repair requests, operational defects, mission logs, and utilization reports help in understanding equipment usage and wear patterns from ships. Maintenance logs and operational reports may be taken from the software tools used in repair yards and shipyards or through the various returns provided by yards on ship refit and repair.
- Machine Learning Based Reliability Assessment Model. In recent years, the utilization of Bayesian Belief Networks has emerged as a powerful approach for risk analysis in complex systems such as gas turbines. Bayesian networks, a subset of probabilistic graphical models, leverage principles of conditional probability to represent and analyse the interdependencies amongst different variables influencing system performance and reliability. The authors, in collaboration with IIT Bombay, have taken a comprehensive study on the application of Bayesian Belief Networks for risk analysis of complex equipment like marine gas turbines. Through the integration of concepts from decision-making, predictive maintenance, and machine learning, the authors aim to develop a sophisticated model that can identify key failure modes, assess their likelihood, and quantify their impact on downtime and performance on equipment, system and even enterprise level process flow.
- Model for Risk and Failure Analysis of a Gas Turbine. The following example illustrates how a component and a subcomponent of the Gas turbine can be modelled for failure and risk analysis. This work has been done by IIT Bombay as part of project NETRA. The same model can be extrapolated to develop a system or enterprise-level risk, failure and reliability analysis tool for better enterpriselevel decision making. The gas turbine equipment was divided into different subassemblies and components for understanding the mutual interaction between subcomponents and their emergent behaviour on the gas turbines. Then each subcomponent was checked in FMD and OREDA software for failure modes. In the software initially, a node (component/sub-assembly/equipment) was defined and then its states were defined as shown in Fig.1.

- Failure mode of an individual system, say the fuel system as above was mapped with the failure mode of the parent equipment i.e. Gas Turbine. The whole layout contains component failure modes and system failure modes. In this example, the model which is created on the failure modes of individual systems, will provide us with a probability of a particular failure mode occurring in a gas turbine. Similarly, this model when extrapolated at the enterprise level, will map the failure modes of individual sub-systems to give the probability of failure of the overall system. It is reiterated here that the model is based on Bayesian theory which gives conditional probability of an event when other events have already occurred. This entire process will happen in real-time, continuously updating itself as new events keep occurring.
- To develop a Bayesian network, Netica software was considered but due to the limitations in the academic version of this software Bayes Fusion software is utilised. As a test case, Failure analysis Networks have been developed for the mentioned systems as follows:-
- Fuel System. The complete fuel system was divided into its individual components and these components were displayed as nodes in the network and failure modes of these components were displayed as the states of a particular node. These failure modes of the components were taken from FMD 91 and OREDA. The same procedure was followed for all the systems.
- Integrating all Systems for GT Failure Analysis. Similarly, all the individual sub-systems of GTs like starting system, Gear Box systems, shafting, fuel systems etc., were mapped into the software. After simulating the depicted networks it was pertinent to combine all these to get a common troubleshooting diagram that can be used to diagnose the GT-level Failure Modes.
Utility of ERP System Backed with RCM Data.
Employing machine learning algorithms to predict reliability based on digital data, current trends, and mission requirements will aid in data-driven decision-making and improve the collective efficiency of the Indian Navy and other services. The maintenance log data from the Naval dockyard will be the integrated with Material Organisation data like time lag in the supply chain, idle time of spare parts in inventory etc., to optimise the demandsupply gap of the engineering spares to achieve overall optimisation in resource planning and utility. The following points will further illustrate how the defence forces will benefit by RCM data-backed ERP system through an example of a naval ship refit management:-
- Decision Support Systems. In the present-day scenario, the timelines of a ship’s refit are planned during the Annual Refit Conference (ARC). Similarly, planning is presumed to exist for the maintenance of war machinery of the Indian Army and Indian Air Force. Based on these planned maintenance routines, scope of work is planned by repair yards and Fore Cast List of spares (FCL) is planned and demanded by material organisation. However, the flow cycle of refits and the operational cycle is not linear. Operational requirements, unavailability of dry docks in repair yards and other administrative bottlenecks add non-linear dimensions to the refit planning. The situation awareness of non-linearity of life cycle management through the machine learning and condition monitoring software tools will aid in optimised decision-making. For example, in present circumstances, if the attenuation of shock mounts of a diesel alternator is showing increasing trend and may merit replacement in the upcoming refit, the Engineer Officer of the ship will intend to place demands of as many shock mounts as are fitted on the alternator. This intent of over demand comes from the lack of predictive failure data of the shock mounts to the Engineer Officer. The same trend extrapolates with the Yard and Material Organisation. Each organisation will intend to overestimate the number of shock mounts required. The underlying issue in this kind of overestimation of demand is in view of the lack of data-driven decision support system. It is therefore imperative to appreciate that decisionmaking at all levels be supported by RCM data.
- Correct Causative Analysis of Defect. The present-day condition monitoring tools being utilised for causative analysis of defects are based on manually logged data, as explained during the introductory part of this paper. This kind of data may not always result in the correct assessment of machinery health. For example, let’s say, the ship is undertaking a hard turn towards port or starboard. While taking a hard turn, the loading on the engine will increase at the same engine rpm but the same reason may not be evident in the diagnostic study of higher lubrication oil temperature. The precise reason of the increase may only be found by regression analysis of lub oil data with all other engine parameters. Therefore, only a comprehensive data-driven machine learning model, as demonstrated above, would be able to predict the comprehensive machinery health.
- Maintenance Cards. Based on the inputs given by this proposed model, Yard can generate automatic maintenance cards for its repair teams thus aiding them with a comprehensive analysis of the failure and the corrective measures to be taken. Similar kinds of maintenance cards may be given to each ship under the refitting authority for optimised maintenance work.
- Resource Allocation. Based on the inputs given by this proposed model, yard can optimise its resource allocation for both workforce and spares as follows:-
- Workforce Management. The workload and skill level required would be predicted by the model so workforce management may be done by using advanced scheduling tools based on the RCM-backed data to assign maintenance tasks to personnel based on availability, skill levels, and workload.1
- Parts and Supplies Coordination. Ensuring that maintenance schedules are aligned with the availability of spare parts and necessary supplies, leveraging the RCM-ERP system help to manage inventory levels effectively.
- HR Assessment. Apart from planning its requirement of the required manpower for a particular refit project, the Yard can also assess the quality of the work undertaken by the yard workers. This assessment would be foolproof because the quality of work done by the yard team will reflect in the machinery reliability number predicted by the model.
- Adaptive Maintenance Scheduling
- Dynamic Scheduling. Utilizing adaptive scheduling systems that can adjust maintenance plans in real-time, based on changing operational conditions and equipment status.
- Risk-Based Prioritization. Prioritizing maintenance tasks based on risk assessments, focusing on equipment that is critical to operations and more prone to failure.
- Feedback and Continuous Improvement
- Performance Monitoring. Continuously monitoring the effectiveness of maintenance schedules and making adjustments based on performance data and feedback.
- Lessons Learned. Documenting lessons learned from maintenance activities to refine predictive models and scheduling algorithms, ensuring continuous improvement.
- Workflow Automation and Process Standardization.
- Automated Workflows. The ERP system will automate workflows, reducing manual intervention and minimizing the risk of errors.
- Standard Operating Procedures. SOPs may be implemented based on continuous trends within the ERP to ensure that all processes are standardised, enhancing consistency and quality.4
- Collaboration and Communication
- Stakeholder Collaboration. An ERP system provides a platform for seamless communication and collaboration among various stakeholders, including shipyard personnel, engineers, and suppliers.
- Document Management. Centralized storage and management of documents related to the refit process, such as blueprints, technical manuals, and compliance reports.
Recommendations
Considering the rapid pace of digitisation of assets as well as processes and the advantages offered by the same as discussed above, through IoTs and digital twins, the following are recommended for the armed forces towards an integrated warfare setup:-
Status of AM in India
- Reliability-Centred Approach. While HR and leadership at different levels or ‘man behind the machine’ is always relevant, the significance of the role and performance of equipment and machinery or the assets used for warfare is irrefutable. These war-fighting assets are expected to meet their respective desired purpose with a defined performance for a defined period of time without failure. ‘Reliability’, which is defined as ‘the degree to which the result of a measurement, calculation, or specification can be depended on, to be accurate’, of an asset plays a vital role at the delivery end of a combat force. Reliability is a direct function of both the design and the maintenance philosophy adopted and is a critical aspect for a fighting force. Thus, a reliability-centred approach for managing the optimal availability and performance of assets is a mandatory requirement of the defence forces. Reliability Centred Maintenance is a maintenance philosophy that assures the desired end state of the assets involved in warfare. However, an RCM philosophy is dependent on accurate data of the assets, both performance and operational related. Therefore, it is pertinent that defence forces acknowledge the importance of RCM for effective life cycle management and high reliability of their assets. 4
- Data Acquisition. Currently, the data related to the performance of the war and related machinery/ equipment is recorded manually in most cases across the defence forces. The maintenance and repair-related data though available is only used locally and ineffectively without any integration and situational awareness. In the haste of task completion and not acknowledging the significance of this data, defence forces face an opportunity loss. Extending the utility of the data over a longer time domain in a structured manner provides institutional retention of knowledge and experience, and aids in accountability of machinery performance. This further equips the user with information that is useful in technological upgradation. Indian Navy, in all new acquisition ships, has upgraded to the state of the art ‘Integrated Platform Management Systems’ with features of integrated data acquisition, which aids in situationally aware combat management within the ship. Extension of the same to the proposed RCM-based integrated DSA philosophy is thus implementable as the next step. The same is, however required to be upgraded on the platforms of older vintage. In the Indian Air Force, the aviation assets including support equipment are sophisticated in nature and are built with data acquisition systems. Integration of the database of these assets in an ERP system is essential to embark on the philosophy of DSA. In the Indian Army, the wide distribution of assets geographically poses a challenge as well as reiterates the necessity of the performance and operational data acquisition, to achieve the desired integration in real-time basis. Thus, all defence services are recommended to adopt to a data acquisition system to capture the operational and maintenance data for effective assets management.
- Assets with Situational Awareness. With a real-time data acquisition from all the assets, the stage is set for ERP-based integrated setup for situationally aware of assets management at multiple levels. Each of the defence forces is required to embark on such a data management system embracing digitisation and interoperability within various organisations of respective service using Big Data Analytics running on an ERP framework towards an enhanced decision support and management system. This would involve creation of an ecosystem that facilitates data acquisition from each asset at sufficient sampling rate, including supply chain data followed by assimilation of this data and converting it to maintenance decisions based on RCM to ensure high reliability of the assets. And this data is shared with other stakeholders at all levels of authority in a secure manner equips operational commanders to arrive at decisions without gaps ensuring optimal utility of the assets in consonance with other assets in the same or connected roles.
- Distributed Situational Awareness. Linking of organisational data, within the respective defence forces and amongst themselves aids in decluttering and streamlining of information overcoming the fog of war. Sound communication networks are essential to achieve this framework of integrated warfare. Indigenous space technology which provided us world-class satellite communication is a strong facilitator in this context. In an integrated framework, the data sharing amongst the sister services is imperative for smooth and dynamic flow of real-time information for effective DSA. The learning curve in the implementation of DSA will commence with the setting up of the required ecosystem followed by progressive implementation from the local assets management to a centralised system of each service and further a data sharing system amongst all the combat forces involved in the integrated warfare towards an effective DSA.
- User Role in Collaborative R&D. All defence forces are required to recognise and acknowledge the significance of reliable war machinery, in light of the rapid technological advancements through collaborative R&D with all stakeholders. OEMs are considered to have a complete know-how of equipment or machine, being the designers. However, the domain expertise of a user in exploiting the equipment is equally valuable in optimising the designs, though not being utilised effectively. Retention of institutional knowledge is a challenge in the organisational set up of a defence force, which involves turnaround of personnel within limited duration of one to three years in a given role. ERP-based data management not only aids in the retention of institutional knowledge but aids in the effective life cycle management of assets. Implementation of advancements in technology in defence forces provides the required edge over adversity, which is directly dependent on the economic prowess of a country. However, effective utilisation of the assets and drawing continuous lessons from the experience can aid in pole vaulting the required technological growth. While the defence production depends on the integrated efforts and collaboration of academia, (like IITs & IISc), designers and the industry, it is the user who has the potential as well as the responsibility to bridge gaps between these agencies. Our country has embarked on a mission to achieve self-reliance in the defence sector as a top priority and our country as such is set to become a developed nation by 2047. Driving the defence technologies is also a prerogative of this ambition because of the inherent potential in the implementation of new technologies in the civil domain. Thus, defence forces are expected to involve in collaborative R&D proactively and assume a leadership role in the development of the nation.
- Resource Sharing. The backbone of an integrated management system is sharing of resources like institutional knowledge in common technological domains, repair yards, advanced field-level testing equipment, satellite communications etc., The resource sharing should cater for overall redundancy within and amongst the defence forces. Optimal peacetime usage by resource sharing with civil applications too in a secure manner will reduce the strain on the government for a developing nation like ours. Common framework and system architectures within and amongst the defence forces will aid in a smooth interface to implement an integrated warfare management system. Multilevel engagement across the services is essential to define and create this common framework.
Conclusion
Situational awareness, reliability-centred maintenance using machine learning, and ERP integration are powerful tool that can significantly enhance material and inventory management in the armed forces. By providing real-time data, predictive analytics, and comprehensive visibility, these approaches enable more accurate inventory management, efficient supply chain operations and improved operational readiness. The Indian Navy, likewise the Indian Army and Indian Airforce, continue to modernise and expand their capabilities, integrating situational awareness, RCM, and ERP systems in its logistical processes will be crucial for maintaining the strategic edge for services in an integrated manner.
DISCLAIMER
The paper is author’s individual scholastic articulation and does not necessarily reflect the views of CENJOWS. The author certifies that the article is original in content, unpublished and it has not been submitted for publication/ web upload elsewhere and that the facts and figures quoted are duly referenced, as needed and are believed to be correct.
*This paper was originally published in CENJOWS SYNERGY Journal [Vol 3 Issue 2] Aug 2024 Edition.
ENDNOTES
- Endsley, M. R. (1995). Toward a Theory of Situation Awareness in Dynamic Systems. Human Factors, 37, 32-64.
- Thakkar, J., & Deshmukh, S. G. (2006). Supply Chain Management in Indian Manufacturing: A Case Study. Production Planning & Control, 17, 512-523.
- Rao, R. (2017). IoT-Based Smart Inventory Management System. International Journal of Advanced Research in Computer Science, 8, 45-50.
- Indian Navy. Annual Report. [online: web] URL: www.indiannavy.nic.in [Accessed 10 June 2024]
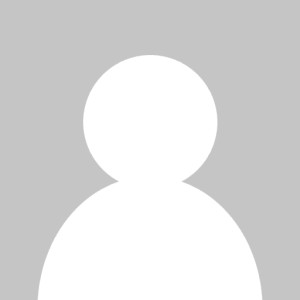
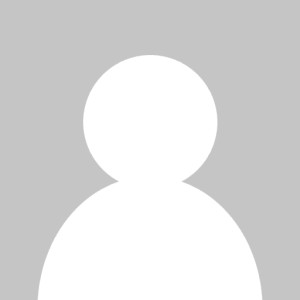
Capt (Dr) MSN Murthy
Capt (Dr) MSN Murthy is an alumnus of, Naval College of Engineering , INS Shivaji and Naval War College. He is an MTech in Thermal and Fluid Engineering and a Doctorate from IIT Bombay with the research topic of ‘Combustion Characteristics in Gas Turbine Engines’. He holds a patent on an innovative heat engine concept called ‘Part Electric Gas Turbine’ through IIT Bombay and has applied for another three patents (under review) on ‘Simple and efficient fuel consumption measuring system for Heat engines’, ‘Recuperator for open architecture GT’, ‘Semi-Closed PEGT for AIP’ through Indian Navy. He is currently heading Centre of Excellence (Marine Engineering), INS Shivaji as Officer-inCharge.
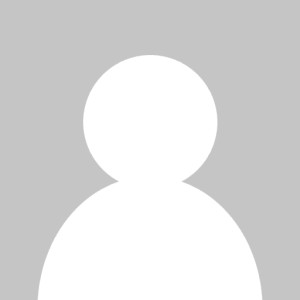
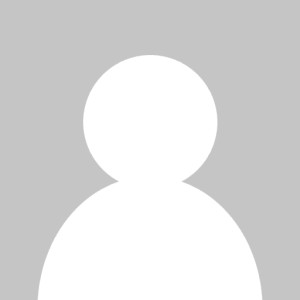
Lt Cdr Apurva Mayank
Lt Cdr Apurva Mayank is an alumnus of Manipal Institute of Technology, Manipal. He is an MTech in Mechanical System Design from IIT Kharagpur and an MBA from Narsee Monjee Institute of Management Studies – Global Access School of Continuing Education. He also holds a Graduate Certificate in Public Policy with specialisation in Defence & Foreign Affairs from Takshashila Institution, Bangaluru. He is currently appointed at Centre of Excellence (Marine Engineering), INS Shivaji and is heading the research verticals related to RCM, BDA, Control Systems and auxiliaries. He is currently pursuing his PhD from IIT Bombay.